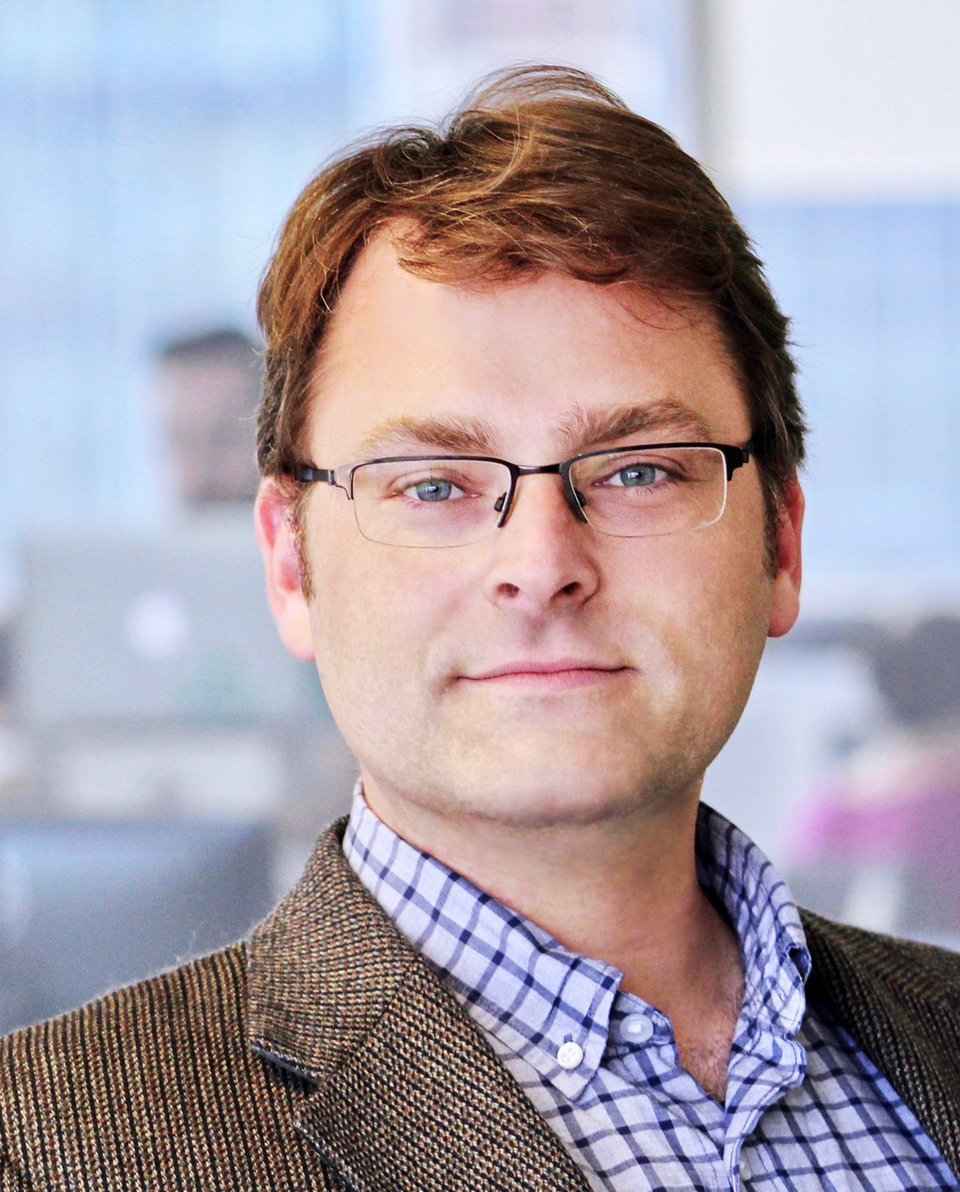
Image: Atomwise CEO and co-founder Abraham Heifets
Image: Atomwise CEO and co-founder Abraham Heifets
Q&A: Atomwise’s journey from discoverer to developer
Artificial intelligence company Atomwise is applying deep learning technology to drug discovery, allowing it to find promising molecules for diseases once considered ‘undruggable’. Following its recent $123m Series B funding round, Abi Millar profiles the growth of the company and finds out what’s in store for 2021 and beyond.
US-based Atomwise is leading the charge in applying artificial intelligence to drug discovery. Since the company was founded in 2012, its platform, AtomNet, has identified a slew of drug candidates for supposedly ‘undruggable’ targets. The company has an extensive range of partnerships with big pharma and small biotechs, along with a number of spin-off companies.
In August, the company announced it had raised $123m in Series B funding, which will be used to scale up all its activities, including its own drug development pipeline. Atomwise CEO and co-founder Abraham Heifets discusses how artificial intelligence is changing the face of drug discovery.
Abi Millar: Atomwise was founded in 2012, with a view to harnessing artificial intelligence for small molecule drug discovery. Can you talk briefly about how you got started?
Abraham Heifets: In 2012, we became the first team to take an AI breakthrough, which was convolutional neural networks, and apply that to drug discovery. This is the same technology that you’ve probably interacted with if you’ve used Siri or Alexa, if you’ve seen a self-driving car, or if you’ve ever uploaded a photo to Facebook and it asks whether you want to tag your friend.
All those technologies use convolutional neural networks – it’s our species’ best technology for image recognition. We were the first team that said we can take those technologies and apply them in the domain of molecular recognition.
Your platform, AtomNet, uses these convolutional neural networks to structure new proteins. Can you explain more about how it works, and why this is so useful within drug discovery?
Let me say a bit about how a drug works. For a drug to be effective it’s got to stick to the disease protein to shut it down and arrest the progress of the disease – you need that potential drug to fit like a key into a lock. But you also want it to be safe and not stick to the thousands of other proteins in the liver or kidney or lung or brain. You need them to bounce off these other proteins that may be very closely related.
You’ve got this underlying question – does it stick to the right thing? So, we frame that as a machine learning problem and we run a prediction, will it stick, do we bother running the physical experiment?
If you think about it, every major industry other than pharma runs their first experiment and tests their first prototypes on the computer. If Airbus is designing a new airplane wing they’ll simulate a thousand before they build one physically. I’m in California right now, and we have a pretty good idea this building will stand up in an earthquake. That’s a very expensive experiment to run. But they simulate it in the computer a thousand times first.
Pharma is the last major industry where you build every prototype physically. You build every one of those molecules, you put it in a physical experiment, you test it to see if it’ll work. So we’re trying to bring the same efficiency to pharma. It’s an exciting time because you don’t get to live through these fundamental shifts in an industry terribly often, but that is what’s going on right now.
To what extent are pharma companies using AI in drug discovery already? What are some of the challenges they face in implementing this kind of technology?
I think everybody’s looking at it, every major pharma company has an effort. We have 778 projects running, and that’s with big pharma companies such as Bayer and Eli Lilly, as well as emerging biotechs, universities and research hospitals around the world. So, there are lots of people across every stage of the pharma research pipeline who are using AI.
The idea of using computational approaches isn’t necessarily new – people have been trying since the late 70s – but previous approaches weren’t able to deliver the accuracy you needed. You needed massive amounts of data, which have only become available recently, and you needed these new computational algorithmic breakthroughs.
Until recently, the state-of-the-art was physical experimentation, and it’s only now we’re seeing that shift to computational.
Your platform has shown great success in finding small molecule hits for previously undruggable targets. What have been some of your key success stories to date?
Let me tell you about one – we’ve published a paper on this with the University of Toledo. There’s a disease, Canavan disease, which is a rare incurable genetic disorder with a high prevalence in Ashkenazi Jews. There’s a molecule in the brain called NAA, with a system in your brain that makes it and a system that breaks it down.
What happens is these kids lose the ability to break it down. Their neurons start to degrade, they stop hitting developmental milestones and they die – it’s a very tragic disease.
There’s some mouse data saying, having lost the ability to clear this molecule, if you slow down production you could bring the system back into balance. So the idea was to slow down production. The problem is, the protein you want to shut down is a tricky protein – it’s near the cell wall so it’s hard to express and purify, and hard to get enough to run the physical experiment. So that was off the table. Nobody had found drug-like molecules, and human intuition had no place to extrapolate from.
Long story short, we’ve overcome some of these problems. We ran a screen of 7.2 million molecules – that’s maybe double the size of a big pharma compound library and we can do that in a couple hours. We tested 60 compounds out of the 7.2 million, and five of those were drug-like molecules. So that’s a case where there’s been a known target for a long time, and nobody’s been able to do anything about it.
Another example is a project we’re doing with our spin-off company, X-37. The PIM3 protein has been shown to be a good target for cancer, but there’s a closely related protein, PIM1, which has associated cardiotoxicity. Lots of pharma companies have worked on PIM3, and there are molecules in the clinic that are PIM inhibitors, but they haven’t been able to achieve the necessary selectivity of PIM3 over PIM1.
We screened 11 billion molecules – thousands of times larger than any big pharma has in its collection – and from that we tested fewer than 500 and were able to find multiple potent and selective compounds. What I like about this is it’s actually a head-to-head comparison. The pharma companies have every physical and computational technique, but the AI unlocked something new.
X-37 is one of a number of spin-off companies you’ve started, with a view to commercialising new molecules. Which lines of research are you most excited about?
The excitement is around the diversity and the breadth. The efficiency improvements from the AI allow us to pursue a range of diverse diseases all in parallel, and even in one disease a range of diverse hypotheses all in parallel. And that’s super exciting because science is messy, right? We don’t know what’s going to work before we do it.
Think about Covid-19. We have 15 different projects for Covid-19 running right now, some of which are working on the viral protein, some of which are working on the human host protein. Rather than doing it sequentially, the AI lets us do them in parallel. Nobody knows which one is going to yield progress a10nd in a sense I don’t think we care – we just want to get to something quickly.
Atomwise raised $123m in its recent Series B funding round. What will you be doing with this financing?
We’re going to scale up in a couple of different ways. The fraction of human proteins that have ever been drugged is around 4%, so there are vast opportunities out there to improve human health and help patients, and we want to expand the technology across more and more molecules.
Having built that, we’re going to be growing our portfolio through partnerships with big pharma companies and biotechs, and also place an increased focus on our own internal discovery projects. So there’ll be continuous scaling in both directions.
What are your next steps as a company? What are some strategic areas of focus for the time being and what are some of your hopes for 2021 and beyond?
Everybody who joins Atomwise does so for the purpose of getting medicines to patients. So we can work backwards from that and see what we need to do next. In the next five years, the hope would be not just to have an asset in the clinic but to have a diverse portfolio.
We’re working on 600 diverse targets across every major therapeutic area, and on multiple different hypotheses for these different diseases. So seeing that whole portfolio move forward into the clinic, that’s the hope. And fundamentally we believe that enormous scale-ups of our technology will enable us to find a cure for many different diseases, not just one.
Q&A: Drug discovery